Shicong Cao is an architect, engineer, and AI enthusiast working at the intersection of artificial intelligence and the built environment. A licensed architect specializing in healthcare design, she has worked with leading firms such as HDR and Heinlewischer, gaining hands-on experience with complex projects that shape the future of healthcare environments. Since 2021, she has focused on leveraging AI and machine learning to transform architectural workflows and urban design processes.
As Founder & CEO of DataDrivenAEC, Shicong is leading the charge to integrate data and AI into architecture, engineering, and construction (AEC). Her work includes pioneering machine learning models to predict the health impacts of the built environment, as demonstrated in her published research “A POI-Based Machine Learning Method in Predicting Health.” She has developed and deployed a range of AI and machine learning solutions
A published researcher in leading computational design conferences, Shicong is committed to promoting data literacy among architects. She is the founder and author of the DataDrivenAEC newsletter, a platform dedicated to exploring the transformative potential of data-driven methods in the AEC industry.
Shicong’s work is driven by a belief that AI and automation can free architects from routine production tasks, enabling them to focus on creating spaces that better serve people. She envisions a future where AEC is a truly user-centered industry, driven by data, empathy, and design excellence.
Below is an initial discussion with Shicong regarding her contributions to AI and architecture. This exchange acts as a lead-in to the BIG Architecture festival in Portorož (May 22–23), where she will take part as a key speaker in the discussion on AI’s role in shaping architecture’s future.
Zala Zia Lenárdič: The use of artificial intelligence and data-driven models enables real-time tracking of spatial usage trends. These models are not only applicable in localized cases, such as traffic planning, but they also provide insights into the future, predicting trends and guiding city development based on collected data.
When questioning the difference between humans and computers, Penrose focused on Gödel’s theorem, which suggests that there are mathematical truths that cannot be proven through computation. For Penrose, the human ability to understand these truths is evidence that humans perform non-computable operations.
With that in mind, how can we integrate human-like non-computable operations—which Penrose might regard as a condition for consciousness—into AI and data-driven city planning? And within a system focused on process optimization, how can we justify decisions that are computationally unprovable? Have you encountered such challenges in your research, where computational results clashed with more intuitive human outcomes?
Shicong Cao: That’s a very good question. We often assume that computational results are inherently inhuman, but in reality, they can be surprisingly aligned with human intuition—even more so than traditional urban planning processes.
For example, in my research, I analyzed key features of a city that best predict residents’ health. Intuitively, we might assume that the number of hospitals would be the strongest predictor. However, statistical models revealed something unexpected: the number of hospitals was not the primary factor. Instead, factors like the number of cafés, accessibility to public transportation, and the quality of public spaces played a more significant role.
These findings align with human intuition in a way—people understand that a livable environment promotes well-being—but they contradict the traditional assumption that hospitals are the most crucial indicator. Data-driven processes like this can uncover deeper truths about city planning, often revealing insights we might not have considered.
“We often assume that computational results are inherently inhuman, but in reality, they can be surprisingly aligned with human intuition—even more so than traditional urban planning processes.”
Tomaž Šimnovec: We know that you’ve conducted extensive research and developed your own models, with articles published on ResearchGate. Would you say that our first question aligns with your research journey? You started in engineering and later transitioned into AI development and computational approaches. How did this shift influence your work?
Shicong Cao: Yes, I began as an engineer—my bachelor’s degree was in engineering—but later, I pursued studies in architecture and health. This interdisciplinary approach led me to consider the health effects of architecture, which involves both subjective and objective elements.
From an architectural standpoint, health considerations can be subjective, but improving health outcomes requires evidence. That’s why I believe combining engineering’s data-driven methods with a human-centered architectural approach makes sense. I conducted research to explore whether these two perspectives could be integrated, and I was surprised by how well the data aligned with real human experiences. Instead of simply planning for more hospitals, the research demonstrated that urban design itself plays a critical role in public health. This is an exciting and relatively new direction for research, with much more to explore.
Tomaž Šimnovec: There’s frequent discussion about weak AI, which can perform a limited set of tasks, versus strong AI, which aims to learn, adapt, and solve complex problems. However, some argue that learning alone isn’t enough to equate AI with understanding—it’s simply more advanced symbol manipulation.
This ties into John Searle’s Chinese Room thought experiment, which suggests that AI can manipulate symbols without actually understanding their meaning. In this scenario, an AI agent might answer questions in perfect Chinese but lack any comprehension of the language itself. Humans, on the other hand, understand the meaning behind symbols but may struggle to articulate the processes they use to do so.
What implications do you think this has for architectural design and the role of AI in working alongside humans?
Shicong Cao: That’s another deep and fascinating question. AI doesn’t need to understand meaning in the human sense to process symbols effectively. Even before generative models like ChatGPT, weak AI was already embedded in many applications.
But do humans truly understand all meanings? I don’t think so. When learning a foreign language, for example, we often begin with minimal cultural context. Translating from one language to another means navigating nuances we may not fully grasp. In that sense, human learning is also a process. Similarly, AI can learn through exposure and refinement.
Working alongside AI can greatly empower architects. Many architects don’t have time to deeply research user behavior in spaces—they’re often occupied with production work. AI can take over time-consuming, lower-value tasks, allowing architects to focus on creative, human-centered aspects of design. By automating certain processes, AI enables designers to engage more deeply with users and develop a richer understanding of spaces.
“AI can take over time-consuming, lower-value tasks, allowing architects to focus on creative, human-centered aspects of design.”
Tomaž Šimnovec: There was a time when smart cities and the Internet of Things (IoT) were seen as the future of urban planning. Do you think this trend still holds, or has the emergence of AI changed the approach?
Shicong Cao: I see IoT as an essential foundation for AI. Buildings and cities now collect vast amounts of data, but many stakeholders—such as building owners—don’t know how to use it effectively. AI can bridge this gap, helping those with limited technical skills derive meaningful insights from data.
Previously, hiring a software engineer to analyze building data was prohibitively expensive. But with AI, in-house teams can leverage data more effectively without requiring specialized expertise. IoT provides the infrastructure for data collection, and AI makes sense of that data.
Tomaž Šimnovec: That’s a great point. I was researching smart architecture and its connection to urban-scale IoT networks. It seems that AI has enhanced, rather than replaced, these ideas. Are there any recent projects—your own or others—that have inspired you in this area?
Shicong Cao: Yes, I’m currently working on an idea that collects user feedback on buildings and processes it using AI, combining this with IoT data to inform long-term building operations and design. Traditionally, once a building is completed, architects have little involvement. I’m curious about how buildings are actually used post-construction and whether improvements can be made.
Architects often receive anecdotal feedback, but this is prone to bias and is rarely documented in a useful way. Meanwhile, many organizations have substantial data but lack the means to analyze it. My work aims to bridge this gap, though it’s still in an early stage.
Additionally, there are many open-source generative AI applications available. I’m interested in how architecture firms use—or don’t use—these tools. Open-source solutions can address concerns like data privacy and AI costs, yet they require some technical knowledge. My previous firm successfully implemented such AI-driven solutions, and I’m curious whether other firms are doing the same.
“Professionals will work with multiple AI ‘co-pilots,’ each specializing in different tasks—one handling documents, another assisting with marketing, and another analyzing data.”
Zala Zia Lenárdič: As AI increasingly integrates into workflows, do you think the role of AI in architecture firms will shift? Will AI function more like a coworker or an assistant?
Shicong Cao: I think rather than a single AI assistant, professionals will work with multiple AI “co-pilots,” each specializing in different tasks—one handling documents, another assisting with marketing, and another analyzing data.
Architecture firms operate differently from tech companies; they don’t emphasize marketing or customer relations in the same way. However, adopting AI-driven approaches could improve their business models. I’m exploring whether AI can enhance architectural firms’ operations and decision-making processes.
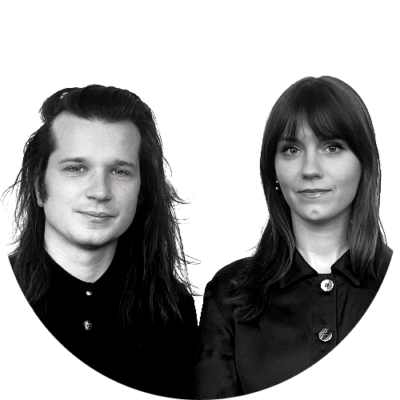
Text: Živa Novak
Curators:
Tomaž Šimnovec & Zala Zia Lenárdič
Portrait & Photos:
NAARO, Namsun Lee, and archive MAA
Powered by
Shicong Cao is an architect, engineer, and AI enthusiast working at the intersection of artificial intelligence and the built environment. A licensed architect specializing in healthcare design, she has worked with leading firms such as HDR and Heinlewischer, gaining hands-on experience with complex projects that shape the future of healthcare environments. Since 2021, she has focused on leveraging AI and machine learning to transform architectural workflows and urban design processes.
As Founder & CEO of DataDrivenAEC, Shicong is leading the charge to integrate data and AI into architecture, engineering, and construction (AEC). Her work includes pioneering machine learning models to predict the health impacts of the built environment, as demonstrated in her published research “A POI-Based Machine Learning Method in Predicting Health.” She has developed and deployed a range of AI and machine learning solutions
A published researcher in leading computational design conferences, Shicong is committed to promoting data literacy among architects. She is the founder and author of the DataDrivenAEC newsletter, a platform dedicated to exploring the transformative potential of data-driven methods in the AEC industry.
Shicong’s work is driven by a belief that AI and automation can free architects from routine production tasks, enabling them to focus on creating spaces that better serve people. She envisions a future where AEC is a truly user-centered industry, driven by data, empathy, and design excellence.
Below is an initial discussion with Shicong regarding her contributions to AI and architecture. This exchange acts as a lead-in to the BIG Architecture festival in Portorož (May 22–23), where she will take part as a key speaker in the discussion on AI’s role in shaping architecture’s future.
Zala Zia Lenárdič: The use of artificial intelligence and data-driven models enables real-time tracking of spatial usage trends. These models are not only applicable in localized cases, such as traffic planning, but they also provide insights into the future, predicting trends and guiding city development based on collected data.
When questioning the difference between humans and computers, Penrose focused on Gödel’s theorem, which suggests that there are mathematical truths that cannot be proven through computation. For Penrose, the human ability to understand these truths is evidence that humans perform non-computable operations.
With that in mind, how can we integrate human-like non-computable operations—which Penrose might regard as a condition for consciousness—into AI and data-driven city planning? And within a system focused on process optimization, how can we justify decisions that are computationally unprovable? Have you encountered such challenges in your research, where computational results clashed with more intuitive human outcomes?
Shicong Cao: That’s a very good question. We often assume that computational results are inherently inhuman, but in reality, they can be surprisingly aligned with human intuition—even more so than traditional urban planning processes.
For example, in my research, I analyzed key features of a city that best predict residents’ health. Intuitively, we might assume that the number of hospitals would be the strongest predictor. However, statistical models revealed something unexpected: the number of hospitals was not the primary factor. Instead, factors like the number of cafés, accessibility to public transportation, and the quality of public spaces played a more significant role.
These findings align with human intuition in a way—people understand that a livable environment promotes well-being—but they contradict the traditional assumption that hospitals are the most crucial indicator. Data-driven processes like this can uncover deeper truths about city planning, often revealing insights we might not have considered.
“We often assume that computational results are inherently inhuman, but in reality, they can be surprisingly aligned with human intuition—even more so than traditional urban planning processes.”
Tomaž Šimnovec: We know that you’ve conducted extensive research and developed your own models, with articles published on ResearchGate. Would you say that our first question aligns with your research journey? You started in engineering and later transitioned into AI development and computational approaches. How did this shift influence your work?
Shicong Cao: Yes, I began as an engineer—my bachelor’s degree was in engineering—but later, I pursued studies in architecture and health. This interdisciplinary approach led me to consider the health effects of architecture, which involves both subjective and objective elements.
From an architectural standpoint, health considerations can be subjective, but improving health outcomes requires evidence. That’s why I believe combining engineering’s data-driven methods with a human-centered architectural approach makes sense. I conducted research to explore whether these two perspectives could be integrated, and I was surprised by how well the data aligned with real human experiences. Instead of simply planning for more hospitals, the research demonstrated that urban design itself plays a critical role in public health. This is an exciting and relatively new direction for research, with much more to explore.
Tomaž Šimnovec: There’s frequent discussion about weak AI, which can perform a limited set of tasks, versus strong AI, which aims to learn, adapt, and solve complex problems. However, some argue that learning alone isn’t enough to equate AI with understanding—it’s simply more advanced symbol manipulation.
This ties into John Searle’s Chinese Room thought experiment, which suggests that AI can manipulate symbols without actually understanding their meaning. In this scenario, an AI agent might answer questions in perfect Chinese but lack any comprehension of the language itself. Humans, on the other hand, understand the meaning behind symbols but may struggle to articulate the processes they use to do so.
What implications do you think this has for architectural design and the role of AI in working alongside humans?
Shicong Cao: That’s another deep and fascinating question. AI doesn’t need to understand meaning in the human sense to process symbols effectively. Even before generative models like ChatGPT, weak AI was already embedded in many applications.
But do humans truly understand all meanings? I don’t think so. When learning a foreign language, for example, we often begin with minimal cultural context. Translating from one language to another means navigating nuances we may not fully grasp. In that sense, human learning is also a process. Similarly, AI can learn through exposure and refinement.
Working alongside AI can greatly empower architects. Many architects don’t have time to deeply research user behavior in spaces—they’re often occupied with production work. AI can take over time-consuming, lower-value tasks, allowing architects to focus on creative, human-centered aspects of design. By automating certain processes, AI enables designers to engage more deeply with users and develop a richer understanding of spaces.
“AI can take over time-consuming, lower-value tasks, allowing architects to focus on creative, human-centered aspects of design.”
Tomaž Šimnovec: There was a time when smart cities and the Internet of Things (IoT) were seen as the future of urban planning. Do you think this trend still holds, or has the emergence of AI changed the approach?
Shicong Cao: I see IoT as an essential foundation for AI. Buildings and cities now collect vast amounts of data, but many stakeholders—such as building owners—don’t know how to use it effectively. AI can bridge this gap, helping those with limited technical skills derive meaningful insights from data.
Previously, hiring a software engineer to analyze building data was prohibitively expensive. But with AI, in-house teams can leverage data more effectively without requiring specialized expertise. IoT provides the infrastructure for data collection, and AI makes sense of that data.
Tomaž Šimnovec: That’s a great point. I was researching smart architecture and its connection to urban-scale IoT networks. It seems that AI has enhanced, rather than replaced, these ideas. Are there any recent projects—your own or others—that have inspired you in this area?
Shicong Cao: Yes, I’m currently working on an idea that collects user feedback on buildings and processes it using AI, combining this with IoT data to inform long-term building operations and design. Traditionally, once a building is completed, architects have little involvement. I’m curious about how buildings are actually used post-construction and whether improvements can be made.
Architects often receive anecdotal feedback, but this is prone to bias and is rarely documented in a useful way. Meanwhile, many organizations have substantial data but lack the means to analyze it. My work aims to bridge this gap, though it’s still in an early stage.
Additionally, there are many open-source generative AI applications available. I’m interested in how architecture firms use—or don’t use—these tools. Open-source solutions can address concerns like data privacy and AI costs, yet they require some technical knowledge. My previous firm successfully implemented such AI-driven solutions, and I’m curious whether other firms are doing the same.
“Professionals will work with multiple AI ‘co-pilots,’ each specializing in different tasks—one handling documents, another assisting with marketing, and another analyzing data.”
Zala Zia Lenárdič: As AI increasingly integrates into workflows, do you think the role of AI in architecture firms will shift? Will AI function more like a coworker or an assistant?
Shicong Cao: I think rather than a single AI assistant, professionals will work with multiple AI “co-pilots,” each specializing in different tasks—one handling documents, another assisting with marketing, and another analyzing data.
Architecture firms operate differently from tech companies; they don’t emphasize marketing or customer relations in the same way. However, adopting AI-driven approaches could improve their business models. I’m exploring whether AI can enhance architectural firms’ operations and decision-making processes.
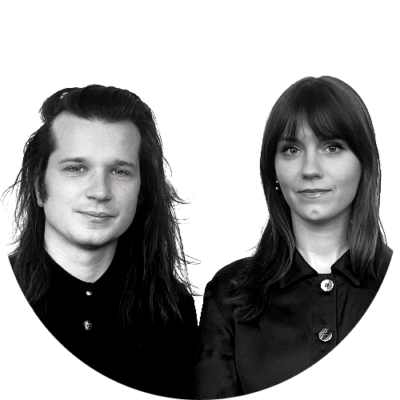
Curators:
Tomaž Šimnovec & Zala Zia Lenárdič
Portrait & Photos:
NAARO, Namsun Lee, and archive MAA